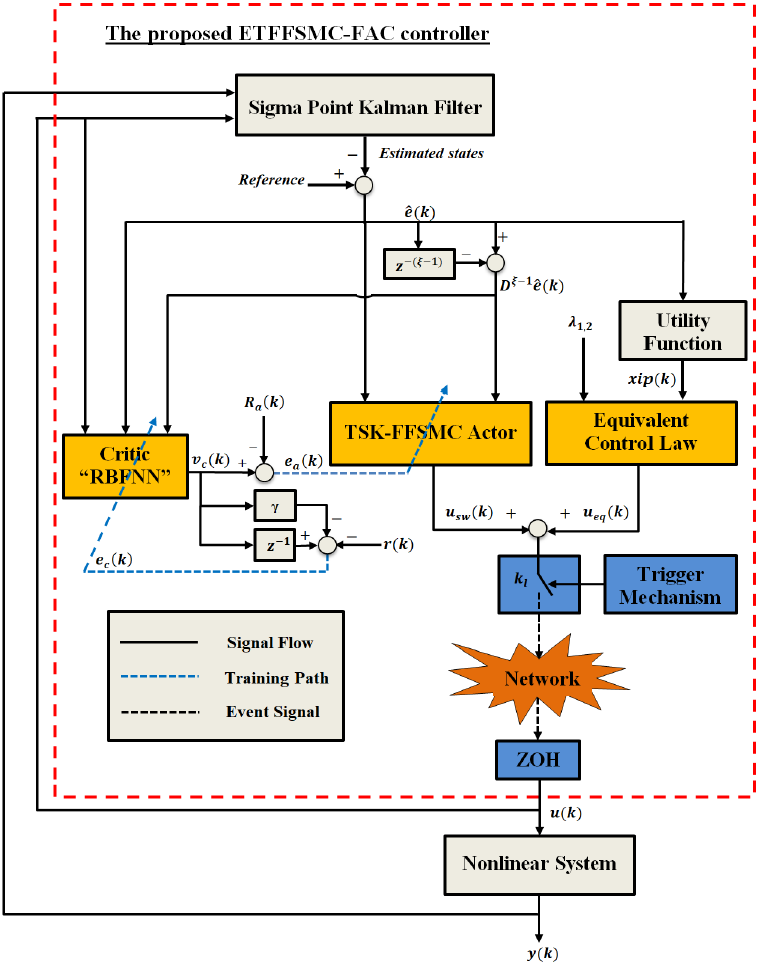
Observer-Based Adaptive Event-Triggered Fractional-Order Sliding Mode Control Using Online Fractional-Order Learning Approach
Abstract
This project introduces an innovative adaptive event-triggered control strategy (ETS) for networked uncertain nonlinear systems with unmeasured states. The proposed method, called ETFFSMC-FAC, combines a fractional-order fuzzy sliding mode controller with a fractional-order actor-critic (FAC) approach. Initially, unmeasured states are estimated using a sigma-point Kalman filter (SKF). Subsequently, a fractional-order sliding mode controller with adaptive ETS is developed to achieve practical sliding mode in the system, free of the Zeno phenomenon. To overcome chattering, a novel fractional-order actor-critic recurrent-like neural network (FAC) is introduced, which properly approximates the switching control stage. The FAC network, based on fuzzy rules defined by a generalized Gaussian function using the Mittag-Leffler function, strikes a balance between minimizing event triggers and ensuring optimal system performance. Additionally, a critic network is employed for value function approximation. Parameter learning laws are optimized using fractional-order Gauss-Newton (FGN), ensuring stability as confirmed by Lyapunov analysis. Finally, the efficacy of the proposed approach is validated through experimentation with a real inverted pendulum (IP).